The Rise of Small and Medium Language Models in AI
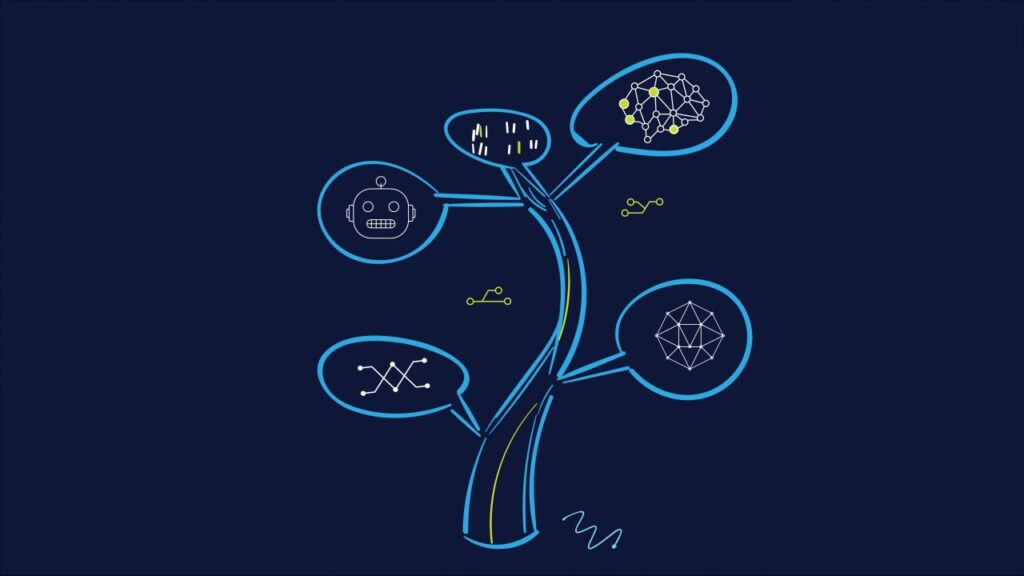
Artificial Intelligence (AI) has seen rapid advancements over the past decade, with large language models (LLMs) such as OpenAI’s GPT-3 and GPT-4 leading the charge. These models, composed of billions of parameters, have demonstrated remarkable capabilities in natural language processing, generating human-like text, and even passing complex exams. However, the trend is shifting towards smaller and more specialized AI models, known as small or medium language models (SLMs). These models are designed to be cheaper, faster, and more efficient, often tailored for specific tasks. This article explores the growing significance of SLMs, their advantages, and their potential impact on various industries.
The Shift from Large to Small Models
Performance Plateau of LLMs
Recent studies indicate that the performance improvements of LLMs are beginning to plateau. For instance, performance comparisons by Vellum and HuggingFace show minimal differences in accuracy among top models like GPT-4, Claude 3 Opus, and Gemini Ultra in specific tasks such as multi-choice questions and reasoning problems. This suggests that simply increasing the size of the model does not necessarily lead to significant performance gains. As a result, researchers and developers are turning their attention to smaller models that can achieve comparable results with fewer resources.
Efficiency and Affordability
One of the primary advantages of SLMs is their efficiency. Smaller models require significantly less computational power, leading to faster processing times and reduced energy consumption. This efficiency translates into lower operational costs, making advanced AI capabilities accessible to a broader range of organizations, including startups and smaller enterprises. For example, models like Mixtral 8x7B and Llama 2 – 70B have shown promising results in reasoning and multi-choice questions, outperforming some of their larger counterparts.
Advantages of Small and Medium Language Models
Cost-Effectiveness
SLMs are considerably more affordable compared to their larger counterparts. The reduced computational and energy demands of these models result in lower costs for training and deployment. This affordability opens up AI possibilities for organizations that previously could not justify the expense of large-scale AI systems.
Flexibility and Specialization
Smaller models offer greater flexibility in integration and application. They can be tailored for specific tasks, such as content categorization, data analysis, and automated customer support. This specialization allows organizations to deploy AI solutions that are precisely aligned with their operational needs, enhancing productivity and efficiency.
Improved Interpretability
Large AI models often operate as “black boxes,” making it difficult for developers to understand how specific outputs are generated. In contrast, smaller models are more interpretable, allowing developers to better understand and adjust the parameters influencing the model’s behavior. This improved interpretability is crucial for creating trustworthy and reliable AI systems.
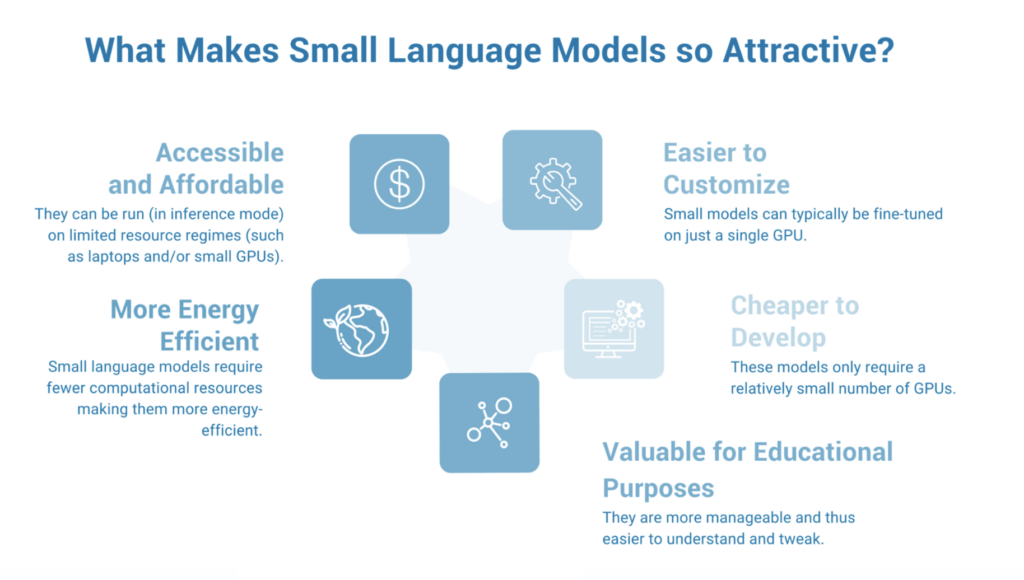
Applications of Small and Medium Language Models
Edge Computing
SLMs are well-suited for edge computing, where data is processed locally on devices rather than relying on centralized cloud infrastructure. This approach reduces latency, enhances data privacy, and improves user experiences. Applications in sectors such as finance, healthcare, and automotive systems benefit from real-time, personalized, and secure AI solutions enabled by SLMs.
Industry-Specific Solutions
SLMs can be customized for industry-specific applications, providing targeted solutions that address unique challenges. For example, in healthcare, SLMs can assist in diagnosing medical conditions by analyzing patient data and medical literature. In finance, they can enhance fraud detection by identifying unusual patterns in transaction data. These specialized models offer significant advantages over general-purpose LLMs, which may lack the nuanced understanding required for specific tasks.
Challenges and Future Directions
Data Quality and Training
While SLMs offer numerous advantages, their performance is heavily dependent on the quality of the training data. High-quality, diverse datasets are essential for training models that can generalize well across different scenarios. Additionally, developing effective training strategies that maximize the capabilities of smaller models remains a critical area of research.
Balancing Size and Capability
Finding the optimal balance between model size and capability is an ongoing challenge. Researchers are exploring various techniques, such as knowledge distillation and transfer learning, to enhance the performance of smaller models without significantly increasing their size. These approaches aim to capture the “minimal ingredients” necessary for intelligent behavior, enabling the development of compact yet powerful AI systems.
Conclusion
The shift towards small and medium language models represents a significant evolution in the field of AI. These models offer a compelling combination of efficiency, affordability, and specialization, making them an attractive alternative to their larger counterparts. As the AI community continues to explore the potential of SLMs, we can expect to see innovative applications and solutions that democratize access to advanced AI capabilities. By focusing on smaller, more interpretable models, researchers and developers are paving the way for a more sustainable and inclusive AI future.